
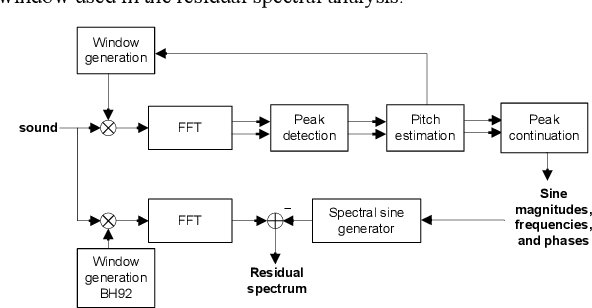
The advantage of having a vocal separation model is that we can use as many audio files The proposed pipeline for training and evaluating an accompanied singer is illustrated in Figure 2. We choose to implement a vocal source separation model with state-of-the-art separation quality (Liu and Yang, 2019) for data preparation. However, public-domain multi-track music data is hard to find. As for the accompanied singer, we need additionally an accompaniment track for each vocal track.
#SINGING VOICE GENERATOR FREE#
Second, for training the free singer, unaccompanied vocal tracks are needed. (a) Singing synthesisįigure 1: Schemes of singing voice generation black Our approach bypasses the step of generating scores by directly generating the mel-spectrogram representation. However, to the best of our knowledge, very few, if any, researches have been done on generating scores of singing voices given an accompaniment. Extensive researches have been done in generating scores of one or several instruments (Hadjeres et al., 2017 Yang et al., 2017 Huang et al., 2019 Payne, 2019). The second step of synthesis is relatively well-established, but the first step of generating a score given an accompaniment is not explored yet. One intuitive approach to achieve this is to first generate a score according to an accompaniment in the symbolic domain and then synthesize the singing voices according to the score. The proposed accompanied singer also represents one of the first attempts to produce singing voice given an accompaniment. This may help establish a universal model based on which extensions can be made. Second, we can more easily use a larger training set to train our model-due to the difficulty in preparing time-aligned scores and lyrics, the training set employed in existing work on SVS usually consists of tens of songs only (Lee et al., 2019a) in contrast, in our case we do not need labeled and aligned data and can therefore use more than hundreds of songs for training. Such freedom may be desirable considering the artistic nature of singing. They enjoy more freedom in the generation output. This work therefore contributes to expanding the “spectrum” (in terms of the strength of conditional signals) of singing voice generation.įirst, while our models are more difficult to train than SVS models, In contrast, the proposed tasks are either Task for generating singing voices, as the target output is well specified by the input. From a technical point of view, we can consider SVS as a
